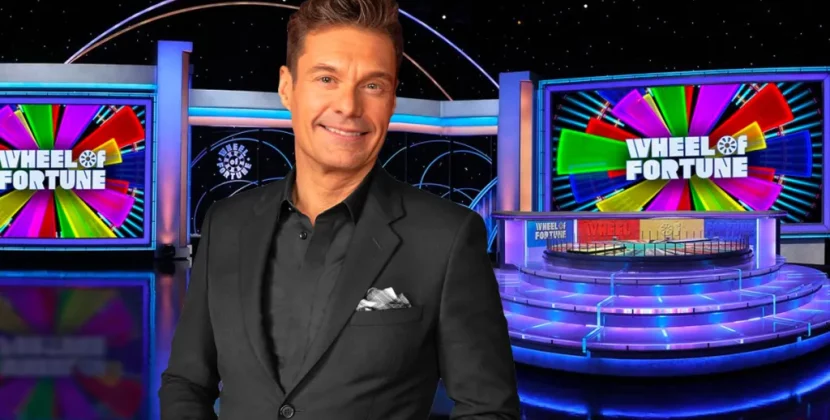
Quantitative finance, a field at the intersection of mathematics, statistics, and finance, has become increasingly reliant on complex models to understand and predict market behavior. These models, ranging from simple linear regressions to intricate neural networks, aim to capture the dynamics of financial markets and aid in decision-making processes. However, as models become more sophisticated, they also become more opaque and challenging to interpret. In this article, we delve into the complexities of quantitative finance models, analyzing their strengths, weaknesses, and the importance of transparency in their application.
The Evolution of Quantitative Finance Models
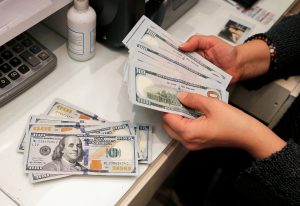
Quantitative finance has evolved significantly over the past few decades, driven by advances in computing power, data availability, and mathematical techniques. Traditional models such as the Black-Scholes option pricing model paved the way for modern quantitative methods. These methods encompass a wide range of approaches, including time series analysis, stochastic calculus, machine learning, and more recently, deep learning.
Strengths of Complex Models
Complex models offer several advantages over simpler ones. They can capture nonlinear relationships, account for interdependencies between variables, and adapt to changing market conditions. For example, neural networks can uncover intricate patterns in data that may be undetectable through traditional statistical methods. Additionally, advanced models like Long Short-Term Memory (LSTM) networks excel at capturing sequential dependencies, making them suitable for time series forecasting in finance.
Weaknesses and Challenges
Despite their strengths, complex models in quantitative finance come with inherent weaknesses and challenges. One major drawback is the risk of overfitting, where a model learns to memorize the training data rather than generalize to new data. This phenomenon can lead to poor performance in real-world scenarios. Moreover, complex models often require large amounts of data and computational resources, making them inaccessible to smaller firms or individuals with limited resources.
Transparency and Interpretability
Transparency and interpretability are crucial aspects of model development and deployment in quantitative finance. Transparency refers to the clarity and openness of a model’s inner workings, while interpretability relates to the ease of understanding and explaining its outputs. In financial markets, where decisions have significant implications, stakeholders require models that they can trust and comprehend.
Analysis Table: Strengths and Weaknesses of Complex Models
Strengths | Weaknesses |
---|---|
Capture nonlinear relationships | Risk of overfitting |
Account for interdependencies between variables | High computational requirements |
Adapt to changing market conditions | Potential for model opacity and lack of transparency |
Uncover intricate patterns in data | Interpretability challenges |
Comparative Table: Traditional vs. Complex Models
Traditional Models | Complex Models |
---|---|
Simple | Sophisticated |
Limited in capturing complex relationships | Able to capture nonlinear relationships |
Easy to interpret | May lack interpretability |
Less computational resources required | High computational requirements |
Black-Scholes option pricing model, CAPM, etc. | Neural networks, LSTM, deep learning models |
Conclusion
Complex models have revolutionized quantitative finance, offering powerful tools for analyzing and predicting financial markets. However, their increased complexity brings forth challenges such as overfitting, computational demands, and interpretability issues. Achieving a balance between sophistication and transparency is essential for effectively leveraging these models in decision-making processes. As quantitative finance continues to evolve, the need for clear, interpretable models remains paramount in ensuring their reliability and trustworthiness.