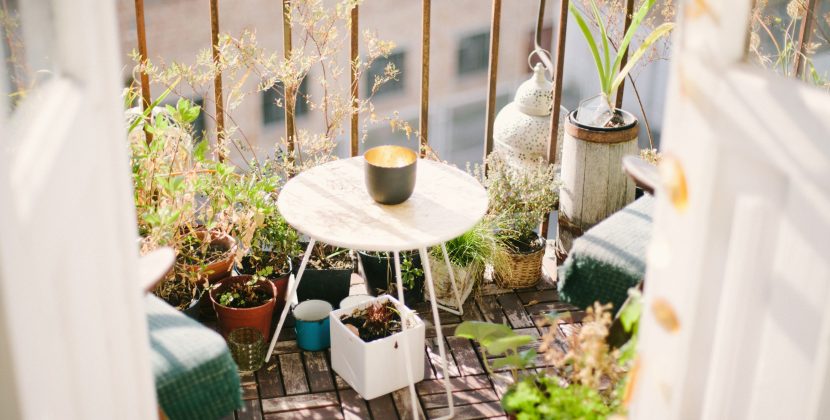
Introduction to AI in Disaster Response
Artificial intelligence (AI) has emerged as a transformative force in disaster response. By leveraging advanced algorithms and data analytics, AI helps predict disasters, coordinate rescue operations, and manage resources efficiently. This article explores the multifaceted role of AI in disaster response, highlighting its impact and potential.
Early Warning Systems: Predicting Disasters Before They Strike
AI-powered early warning systems analyze vast amounts of data from various sources, such as weather patterns, seismic activity, and social media feeds. These systems can predict natural disasters like hurricanes, earthquakes, and floods with remarkable accuracy, giving communities precious time to prepare and evacuate.
Example: The AI-driven early warning system developed by IBM has successfully predicted floods in India, providing timely alerts that helped save thousands of lives.
AI in Search and Rescue Operations
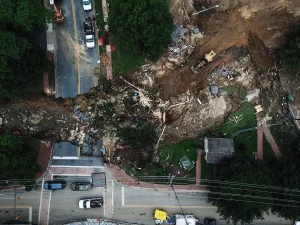
During disasters, AI plays a crucial role in search and rescue missions. AI-powered drones and robots can navigate through dangerous terrains, locating survivors and delivering essential supplies. Machine learning algorithms analyze images and sensor data to identify people in need of help.
Example: In the aftermath of the 2019 Australian bushfires, AI-equipped drones were used to find trapped animals and assess damage in inaccessible areas.
Managing Resources and Logistics with AI
Effective disaster response requires efficient resource allocation and logistics management. AI systems optimize the distribution of medical supplies, food, and shelter, ensuring that aid reaches the affected areas quickly and efficiently.
Example: The United Nations World Food Programmed uses AI to streamline its supply chain, ensuring that food and resources are delivered promptly during emergencies.
Case Studies: Real-World Applications of AI in Disaster Response
Case Study 1: AI in Earthquake Response
In Japan, AI systems predict aftershocks following major earthquakes, helping to coordinate evacuation efforts and allocate resources where they are most needed.
Case Study 2: AI in Hurricane Relief
After Hurricane Harvey, AI algorithms were used to analyze satellite imagery and assess damage, enabling quicker response and recovery efforts.
Challenges and Ethical Considerations
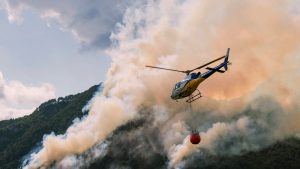
While AI offers immense potential in disaster response, it also raises ethical and practical challenges. Issues such as data privacy, algorithmic bias, and the need for human oversight are critical considerations.
Key Challenge: Ensuring that AI systems are transparent and accountable to avoid biases that could disproportionately affect vulnerable populations.
The Future of AI in Disaster Response
The future of AI in disaster response looks promising, with advancements in machine learning, data analytics, and robotics set to enhance our capabilities further. Integrating AI with other technologies like the Internet of Things (IoT) and 5G networks will create more robust and responsive systems.
Future Trend: The development of AI-powered predictive models that can simulate various disaster scenarios, helping governments and organizations to better prepare for potential crises.
Visual Table: Key Applications of AI in Disaster Response
Application | Description | Example |
---|---|---|
Early Warning Systems | Predict natural disasters using data analytics and machine learning. | IBM’s flood prediction system in India |
Search and Rescue Operations | Use AI-powered drones and robots to locate survivors and deliver supplies. | Drones in Australian bushfires |
Resource Management | Optimize distribution of medical supplies, food, and shelter. | UN World Food Programme supply chain |
Damage Assessment | Analyze satellite imagery and sensor data to assess disaster impact. | AI in Hurricane Harvey relief |
Predictive Modeling | Simulate disaster scenarios to improve preparedness. | Future AI predictive models |
Comparative Table: Traditional vs. AI-Enhanced Disaster Response
Aspect | Traditional Response | AI-Enhanced Response |
---|---|---|
Prediction Accuracy | Limited, often based on historical data | High, using real-time data and machine learning algorithms |
Resource Allocation | Manual and time-consuming | Automated and optimized through AI systems |
Search and Rescue | Human-led, slower, and riskier | AI-powered drones and robots, faster and safer |
Damage Assessment | Ground surveys and manual reporting | AI analysis of satellite imagery and sensor data |
Response Time | Slower, dependent on human coordination | Faster, driven by AI algorithms and real-time data processing |
Conclusion
AI is revolutionizing disaster response, offering innovative solutions that enhance efficiency and save lives. From early warning systems to resource management and search and rescue operations, the integration of AI in emergency management holds the promise of a safer, more resilient future.