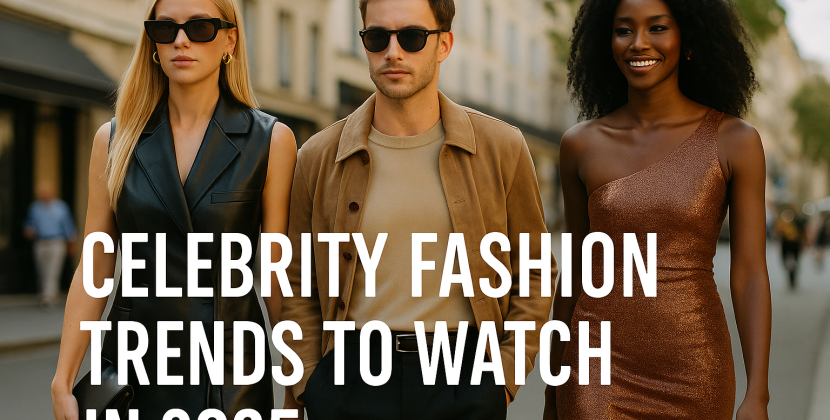
Introduction
The advancement of generative language models like ChatGPT has brought with it increased attention to the biases inherent within these systems. This article aims to investigate the challenges and risks associated with biases in large-scale language models like ChatGPT. We will explore the origins of biases, ethical concerns, potential opportunities to mitigate biases, and the implications of deploying these models in various applications. Our goal is to foster a thoughtful dialogue within the AI community, encouraging researchers and developers to reflect on the role of biases in generative language models and the pursuit of ethical AI.
Defining Bias in Generative Language Models
We delve into the factors contributing to biases in large language models, such as ChatGPT. These biases can stem from the training data, algorithms, labeling and annotation process, product design decisions, and policy choices. Understanding these factors is crucial in addressing biases effectively.
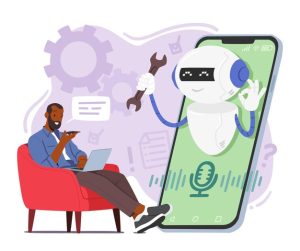
Types of Biases in Large Language Models
Various types of biases can manifest in large language models due to their training data and inherent characteristics. We explore demographic biases, cultural biases, linguistic biases, temporal biases, confirmation biases, and ideological and political biases, discussing their implications on model behavior and outputs.
The Ethical Implications of Bias
Biases in AI systems can have ethical consequences, perpetuating stereotypes, and promoting unfair treatment. We examine the ethical concerns arising from the unintended consequences of biased model outputs and the responsibilities of AI developers in mitigating these biases.
Mitigating Biases in Language Models
We analyze potential opportunities to mitigate biases in large language models, exploring the challenges in achieving unbiased AI. While some biases may be inevitable due to the training data, we discuss techniques like adversarial training and dataset curation to reduce their impact.
Deploying Language Models Responsibly
Considering the widespread applications of language models like ChatGPT, we discuss the ethical considerations involved in their deployment, emphasizing the importance of transparency and responsible AI development.
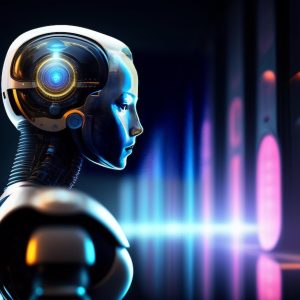
Current Approaches to Identifying and Mitigating Biases
We review existing approaches to identify, quantify, and mitigate biases in language models. Collaborative efforts between researchers, practitioners, and policymakers are crucial in developing equitable, transparent, and responsible AI systems.
Conclusion
Addressing biases in large language models is a complex and multifaceted challenge. By stimulating thoughtful discussions and promoting ethical AI development, we aim to pave the way for more responsible and unbiased AI solutions. As the AI community continues to evolve, it is essential to prioritize fairness and inclusivity in language model development to ensure beneficial outcomes while minimizing potential harm.