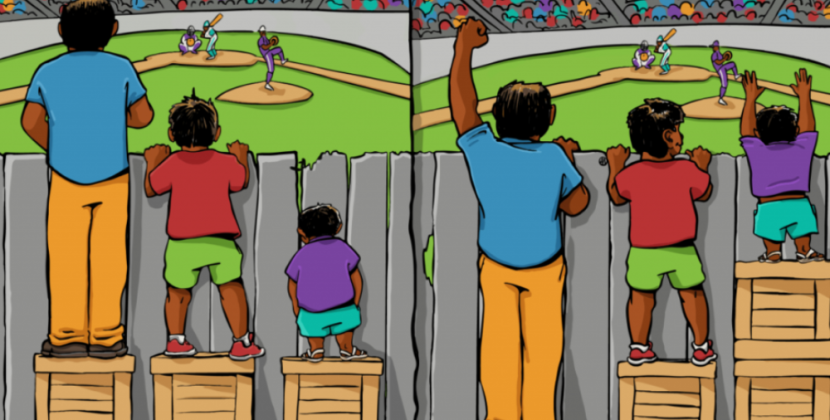
Introduction
Personalized product recommendations have become indispensable for online platforms to enhance user experience and increase customer engagement. By tailoring suggestions based on consumers’ preferences, platforms can offer relevant and enticing options, leading to improved customer satisfaction and higher conversion rates. However, the collection and use of personal data for generating personalized recommendations raise privacy concerns among users.
To address these privacy challenges, we propose an innovative approach that leverages differential privacy to design privacy-preserving personalized recommendation policies. By introducing randomness into the recommendation outcomes, we can thwart inference attacks and protect consumers’ sensitive information. This article aims to provide an overview of our research on the theoretical model and its economic implications, offering valuable insights for both practitioners and regulators.
Theoretical Model: A Coarse-Grained Threshold Policy
In our theoretical model, the recommendation policy selects products to suggest based on consumers’ preference rankings, which are learned from personal data such as cookies. To maintain privacy, we introduce differential privacy into the process, ensuring that the recommendation outcomes do not reveal specific individuals’ preferences. The optimal policy, in this case, is a coarse-grained threshold policy.
Under the threshold policy, products are randomly selected for recommendation, and a subset of these products is assigned higher recommendation probabilities than the rest. The selection of the priority subset is determined by applying a threshold to the consumer’s preference ranking. This randomization of recommendations prevents adversaries from inferring individual preferences, thus safeguarding consumer privacy.
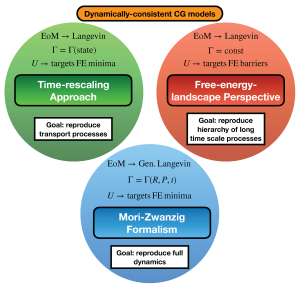
Economic Implications: Privacy Protection and Consumer Surplus
We also explore the economic consequences of privacy protection in personalized recommendations. When product prices are predetermined, implementing privacy protection reduces consumer surplus due to the diminished match value of the recommended products. This is because the randomness introduced in the recommendation process may lead to less accurate suggestions, reducing the utility consumers derive from their purchases.
However, when retailers optimally set prices, the impact of privacy protection on consumer surplus becomes non-monotonic. Here, there is a trade-off between recommendation accuracy and price inflation. While privacy-preserving policies might decrease accuracy, leading to some reduction in consumer surplus, it also curtails the potential for price discrimination and ensures fairer pricing practices.
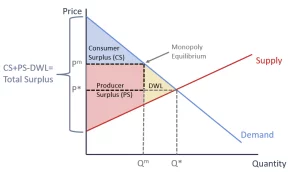
Managerial and Regulatory Implications
For practitioners and businesses seeking to design personalized recommendation strategies, our study offers valuable insights. By adopting differential privacy techniques, platforms can strike a balance between personalization and privacy, providing users with tailored recommendations while safeguarding their sensitive information.
Regulators can also benefit from our research, gaining a deeper understanding of the economic repercussions of privacy protection. This understanding is crucial in shaping privacy regulations that strike a balance between encouraging personalized services and safeguarding user privacy.
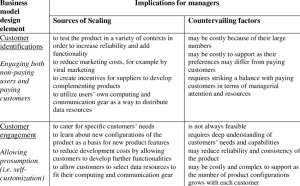
Conclusion
Privacy-preserving personalized recommender systems, empowered by differential privacy, present a promising solution to address privacy concerns in online platforms. By adopting a coarse-grained threshold policy, businesses can protect consumer privacy while offering tailored recommendations.
Our study highlights the economic implications of privacy protection and emphasizes the importance of striking a balance between recommendation accuracy and privacy preservation. As technology and regulations continue to evolve, the adoption of privacy-preserving strategies will play a pivotal role in shaping the future of personalized recommendations in the digital landscape.