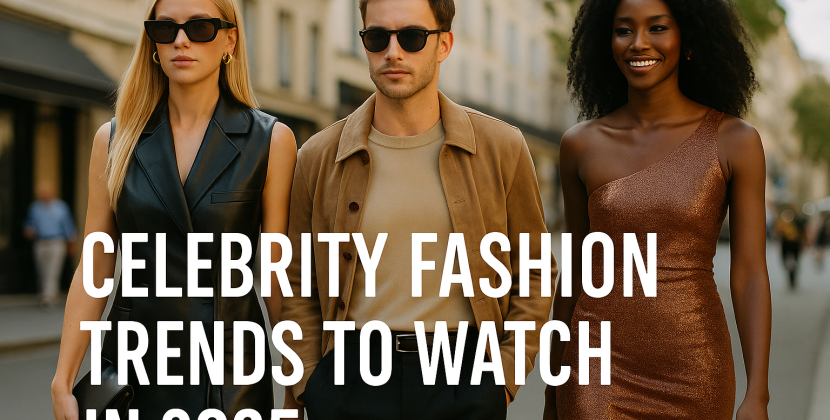
Introduction
The development of AI has enabled automation of complex processes., An intriguing area of research in AI is MARL., The MARL team is dedicated to advancing the field of multi-agent learning., Artificial intelligence’s capacity to innovate multiple sectors is immense, ranging from robotics and self-driving cars to banking and medical care through joint effort and rivalry among AI frameworks.
The Essence of MARL
The heart of MARL is built upon the principles of reinforcement learning (RL), a subfield of machine learning that enables a reasoning being to mature through involvement with its surroundings and acceptance of incentives or reproof due to its endeavors. The agent’s primary goal is to increase cumulative rewards by refining its decision-making policy through exploration and knowledge acquired from past encounters. Interactive AI agents contribute to intricate learning dynamics.,
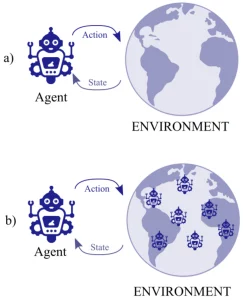
Balancing Cooperation and Competition
Marrying cooperation with competition poses a significant challenge in MARL. Agents must collaborate when covering a sizable area using a swarm of drones. Sometimes, agents may prioritize disparate objectives, leading to competitive tension or resource competition., as observed in financial dealings or robotic vehicle operation.
Researchers have presented diverse solutions to deal with these difficulties. A method that combines focused learning with distributed execution includes… A single central controller is utilized during training to oversee the joint instruction of agents using the global state of the environment and their teamwork actions. While executing, each agent bases its choices on nearby observations and acquired policies independently. Training enables agents to develop joint tactics while preserving execution versatility and durability.
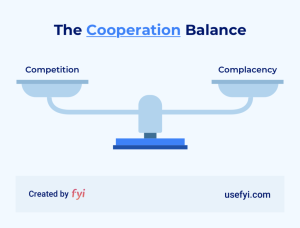
Enhancing Communication Protocols
MARL investigations are centering on creating methods that let agents share information and align their behaviors better. Communication and signal transmission between agents during learning enhance their capacity to forecast actions and modify behavior. Complex cooperative and competitive strategies arise from this, making individual learning insufficient.
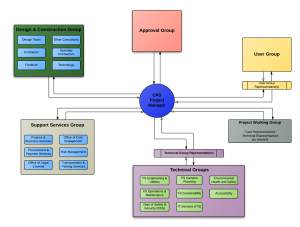
Empowering Efficiency and Generalization
Exploring transfer learning and meta-learning can boost the efficiency and accuracy of MARL algorithms. Leveraging previously gained expertise speeds up learning in a similar context. In contrast, meta-learning focuses on training agents to promptly adapt to novel assignments or settings with minimum additional instruction. The two methodologies demonstrate outstanding potential in fostering AI systems’ learning abilities in sophisticated, adaptive, and unforeseen multi-agent situations.
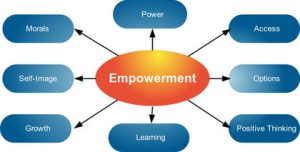
The Future of MARL
The ongoing development of MARL portends significant societal consequences. MARL’s capabilities extend far beyond traffic management and financial market operations., Innovative approaches to multi-agent learning through AI can unlock new potential for resolving global challenges.
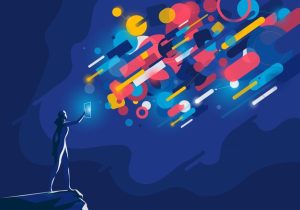
Conclusion
The groundbreaking approach of Multi-Agent Reinforcement Learning enables collaboration and rivalry among AI agents. The synergy of algorithm refinement, communication protocol enhancement, and meta-learning integration could reshape numerous sectors. Advancements in the field will enable AI to tackle complex challenges, leading to a promising outlook for MARL.