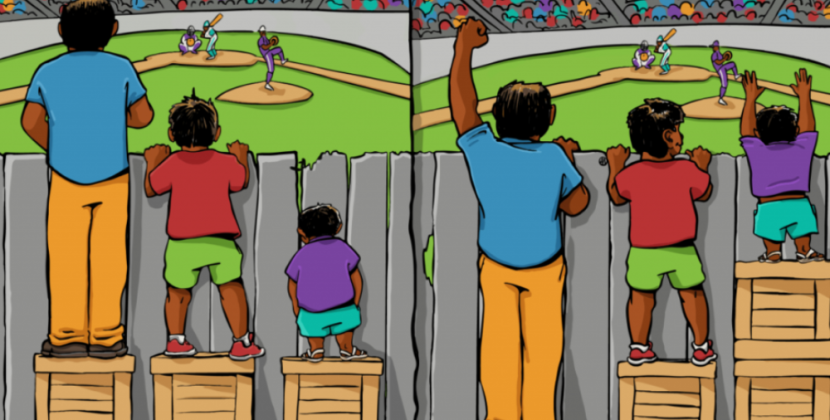
Introduction
In the ever-evolving landscape of artificial intelligence, ChatGPT and progressive networks have emerged as powerful tools for continuous learning through transfer learning. Transfer learning allows models like ChatGPT to leverage knowledge gained from one domain and apply it to new tasks, enhancing their performance and versatility. In this article, we will explore how ChatGPT and progressive networks work hand in hand, enabling continuous learning with practical applications across various domains.
Understanding ChatGPT and Transfer Learning
ChatGPT, developed by OpenAI, is an advanced language model that uses the power of deep learning and vast amounts of text data to generate coherent and realistic text. It has been trained on diverse sources like articles, books, and Wikipedia, making it a comprehensive language-generating AI.
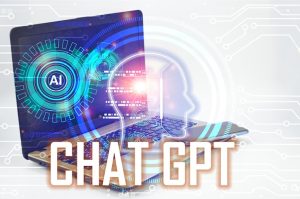
Transfer learning is a machine learning technique that involves taking knowledge learned from a source domain and applying it to a target domain. In the context of ChatGPT, transfer learning allows the model to be pretrained on a large dataset (source domain) and then fine-tuned for specific tasks (target domain). This process enables ChatGPT to adapt quickly to new tasks with minimal additional training.
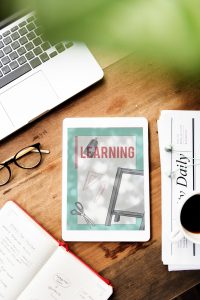
The Promise of Progressive Networks
Progressive networks are a novel model architecture designed explicitly to support transfer learning across sequences of tasks. Unlike traditional finetuning methods that discard previously learned knowledge, progressive networks retain a pool of pretrained models throughout training. These models are equipped with lateral connections that allow them to extract useful features from previous tasks.
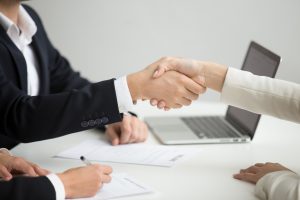
The lateral connections in progressive networks enable a richer compositionality, making prior knowledge non-transient and easily integratable at each layer of the feature hierarchy. Additionally, progressive networks can add new capacity alongside pretrained networks, giving them the flexibility to learn new information while still reusing old computations. This design ensures that progressive networks accumulate experiences over time and are immune to catastrophic forgetting, making them ideal for continuous or lifelong learning scenarios.
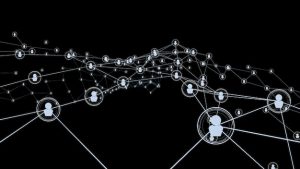
Applications of Progressive Networks in Reinforcement Learning
Progressive networks find particular relevance in deep reinforcement learning, where agents learn to solve a series of tasks in sequence. Each task corresponds to a Markov Decision Process (MDP), and progressive networks can adapt to each MDP by creating a new column for each new task. The lateral connections enable the reuse of features from previous columns, enhancing convergence speed and performance in complex reinforcement learning domains.
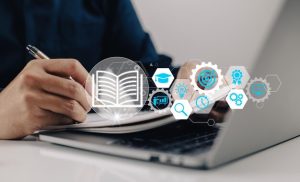
Adapters are an essential component of progressive networks in practice. These non-linear lateral connections play a dual role in improving initial conditioning and performing dimensionality reduction. By using adapters, progressive networks can effectively handle tasks with varying degrees of complexity, making them more adaptable to a wide range of real-world problems.
Benefits and Limitations of Progressive Networks
One significant advantage of progressive networks is that they do not destroy previously learned features during training. This unique characteristic allows for detailed analysis of where and how transfer occurs across tasks. Methods like Average Perturbation Sensitivity (APS) and Average Fisher Sensitivity (AFS) provide insights into the contributions of source columns to the target task. These analyses show that progressive networks enable efficient transfer without catastrophic forgetting, making them a valuable asset in continual learning scenarios.
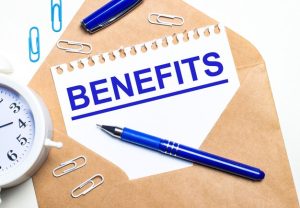
However, a limitation of progressive networks lies in their parameter growth with the number of tasks. As more columns are added, the number of parameters increases, potentially leading to scalability challenges. Addressing parameter growth through techniques like pruning or online compression can help mitigate this issue.
Real-World Applications
The combination of ChatGPT and progressive networks opens up a world of possibilities for real-world applications. Let’s explore a few examples:
- Personalized Customer Service: Progressive networks can be used to enhance chatbots like ChatGPT to provide personalized and context-aware customer support. By leveraging knowledge from past interactions, chatbots can better understand user queries and respond more effectively.
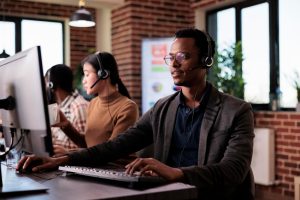
- Medical Diagnosis and Treatment: ChatGPT, when integrated with progressive networks, can become a valuable tool for medical professionals. It can assist in diagnosing patients by learning from vast medical literature and case studies, ultimately leading to improved healthcare outcomes.
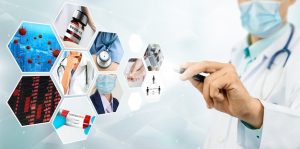
- Education and E-Learning: Progressive networks can revolutionize the field of education by providing adaptive and personalized learning experiences. By understanding individual student needs and learning patterns, the system can deliver tailored educational content and support.
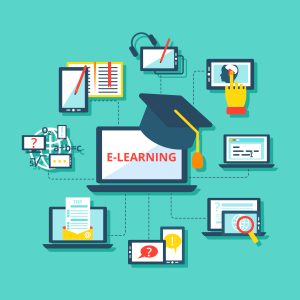
- Robotics and Automation: In robotics, progressive networks can facilitate knowledge transfer between different robotic tasks. This enables robots to learn from various environments and experiences, improving their adaptability and performance.
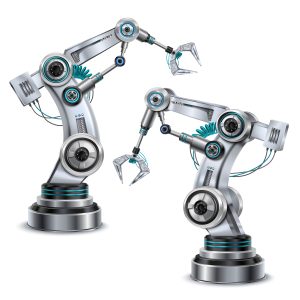
Conclusion
The combination of ChatGPT and progressive networks marks a significant advancement in the field of continuous learning and transfer learning. These powerful tools enable models to retain and build upon previously learned knowledge, making them highly versatile and adaptable to new tasks. As we continue to explore the potential of these technologies, we can look forward to more innovative applications that positively impact various industries and domains. Continuous learning is no longer a distant vision; it’s a reality, thanks to the collaboration of ChatGPT and progressive networks.