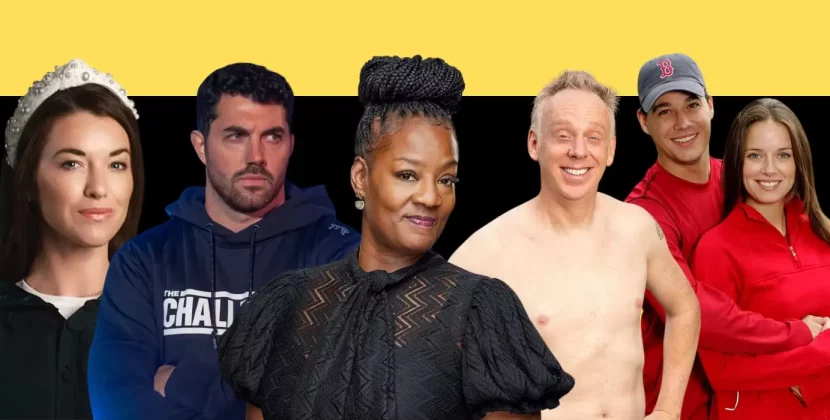
Artificial intelligence (AI) is transforming various industries, and one of the key areas where it is making a substantial impact is in credit risk assessment. As financial institutions seek to improve accuracy, reduce risks, and enhance decision-making processes, AI-driven technologies are becoming essential tools for analyzing creditworthiness. This article explores the role of AI in enhancing credit risk assessment, its benefits, and how it compares with traditional methods.
Introduction to Credit Risk Assessment
Credit risk assessment is the process of evaluating a borrower’s ability to repay a loan or meet other financial obligations. Financial institutions, such as banks, credit unions, and lending companies, use this evaluation to determine whether they should extend credit to a particular individual or business. The main factors typically analyzed include the borrower’s credit history, income, financial stability, and other relevant information.
Traditionally, this assessment has relied on historical data and manual processes, where analysts review financial statements and apply predefined criteria. However, with the increasing complexity of global financial markets, this traditional method has shown its limitations, especially in terms of scalability, accuracy, and speed.
How AI is Changing Credit Risk Assessment
Artificial intelligence, particularly machine learning (ML) and data analytics, offers new possibilities for improving credit risk assessment. By leveraging large datasets and complex algorithms, AI can analyze patterns and identify correlations that may not be obvious to human analysts.
Here are some ways AI is transforming credit risk assessment:
1. Improved Accuracy
One of the biggest advantages of AI in credit risk assessment is its ability to improve the accuracy of predictions. Machine learning models can process vast amounts of data from various sources, including social media, transaction history, and other behavioral data. These models can identify patterns that are often missed by traditional methods, allowing lenders to make more informed decisions.
2. Enhanced Speed and Efficiency
AI-driven credit risk assessment systems can process large datasets in a fraction of the time it takes for human analysts to do so. This speed allows for faster decision-making, which is particularly important in today’s fast-paced financial environment. Lenders can approve or deny loans more quickly, improving customer satisfaction and operational efficiency.
3. Reduced Human Bias
Traditional credit risk assessment models are often subject to human bias, which can lead to unfair lending practices. AI systems, when properly trained and implemented, can reduce these biases by focusing solely on data-driven insights. However, it is essential to ensure that the data fed into AI systems is diverse and unbiased to avoid perpetuating existing biases.
4. Real-Time Monitoring and Dynamic Scoring
AI can enable real-time credit risk monitoring, providing financial institutions with up-to-date insights into a borrower’s creditworthiness. Unlike traditional credit scores, which are static and updated periodically, AI models can provide dynamic scoring that adjusts based on a borrower’s financial behavior and market conditions.
5. Incorporation of Alternative Data
AI allows for the inclusion of alternative data sources, such as utility bills, mobile payments, and even social media activity, which are not typically considered in traditional credit assessments. This is particularly beneficial for borrowers with limited or no credit history, providing lenders with a more comprehensive view of their creditworthiness.
AI vs. Traditional Credit Risk Assessment
Traditional Credit Risk Assessment
Traditional credit risk assessment methods rely on historical financial data and credit scores. Analysts use this information to predict a borrower’s ability to repay a loan. While these methods have been effective for decades, they are not without limitations.
- Data Scope: Traditional methods often rely on limited datasets, such as credit reports and financial statements. This narrow scope can result in incomplete assessments.
- Processing Time: Manual credit risk assessments are time-consuming and can take days or even weeks to complete.
- Human Judgment: While human judgment is important, it can introduce bias into the decision-making process.
AI-Powered Credit Risk Assessment
AI-powered credit risk assessment uses advanced algorithms and machine learning to analyze vast amounts of data in real-time. These methods offer several key advantages over traditional approaches:
- Data Scope: AI systems can analyze large and diverse datasets, including non-traditional data sources like social media activity, transaction history, and more.
- Processing Time: AI can process credit assessments in real-time or within minutes, significantly improving efficiency.
- Data-Driven Decisions: AI eliminates much of the human bias that may influence traditional assessments, providing objective, data-driven results.
Comparative Analysis Table
Criteria | Traditional Credit Risk Assessment | AI-Powered Credit Risk Assessment |
---|---|---|
Data Scope | Limited to historical financial data | Incorporates large, diverse datasets |
Processing Time | Manual, time-consuming (days to weeks) | Real-time or within minutes |
Human Bias | High potential for bias | Reduces bias with data-driven decisions |
Alternative Data Usage | Limited, mostly financial data | Uses alternative data (e.g., social media, utility bills) |
Real-Time Monitoring | No | Yes |
Dynamic Credit Scoring | Static scores updated periodically | Dynamic, adjusts based on real-time data |
Benefits of AI in Credit Risk Assessment
1. Increased Access to Credit
One of the primary benefits of AI in credit risk assessment is its ability to expand access to credit. By considering alternative data sources, AI models can provide credit to individuals who may not qualify under traditional assessment models, such as those with little or no credit history. This creates opportunities for financial inclusion, particularly in underserved markets.
2. Better Risk Management
AI improves risk management by providing more accurate and up-to-date assessments of a borrower’s creditworthiness. Lenders can make better decisions, reducing the likelihood of defaults and minimizing financial losses. Real-time monitoring also enables lenders to adjust credit limits or terms if a borrower’s financial situation changes.
3. Cost Reduction
AI-driven credit risk assessment reduces the need for manual processing, lowering operational costs for financial institutions. Automated systems require less human intervention, freeing up resources for other tasks. The reduction in processing time also means that lenders can serve more customers in less time.
Challenges of AI in Credit Risk Assessment
Despite its numerous benefits, the adoption of AI in credit risk assessment is not without challenges:
1. Data Privacy and Security
The use of AI involves analyzing large amounts of data, including sensitive personal and financial information. Ensuring data privacy and security is critical, as breaches can lead to severe legal and financial consequences for both lenders and borrowers.
2. Bias in AI Models
While AI has the potential to reduce human bias, it is not immune to bias itself. If the data used to train AI models is biased, the results can perpetuate those biases. This could lead to unfair credit decisions, particularly for certain demographic groups.
3. Regulatory Compliance
Financial institutions must comply with various regulations related to lending practices and data privacy. The use of AI in credit risk assessment raises questions about transparency and accountability. Regulators may need to update existing rules to ensure that AI models are used fairly and ethically.
Future of AI in Credit Risk Assessment
The future of AI in credit risk assessment looks promising as technology continues to evolve. As AI algorithms become more sophisticated, they will be able to provide even more accurate and personalized credit assessments. Additionally, the incorporation of blockchain technology could further enhance transparency and security in the credit assessment process.
Lenders will continue to adopt AI-driven credit risk assessment tools to improve decision-making, reduce risks, and enhance customer satisfaction. However, it is crucial to address the challenges of bias, data privacy, and regulatory compliance to ensure that AI is used responsibly in the financial sector.
Analysis Table
Advantages of AI in Credit Risk Assessment | Challenges of AI in Credit Risk Assessment |
---|---|
Improved accuracy and speed | Potential bias in AI models |
Reduced human bias | Data privacy and security concerns |
Real-time monitoring and dynamic scoring | Compliance with regulations |
Inclusion of alternative data | Lack of transparency in AI decision-making |
Increased access to credit | Dependence on quality data inputs |
Conclusion
AI is revolutionizing credit risk assessment by offering more accurate, efficient, and data-driven insights compared to traditional methods. By leveraging large datasets and alternative data sources, AI can expand access to credit, reduce bias, and improve risk management. However, to fully realize the potential of AI in credit risk assessment, it is essential to address challenges related to data privacy, security, and regulatory compliance. As AI technology continues to advance, it will play an increasingly important role in the financial industry, providing lenders with the tools they need to make smarter, faster, and fairer lending decisions.