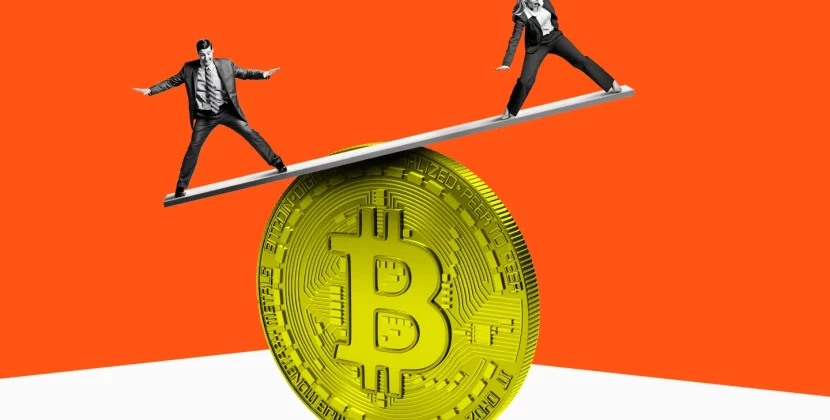
Fraud detection in finance has seen significant transformations over the years, especially with the growth of technology and the increasing complexity of financial systems. As the financial landscape evolves, so do the techniques and tools for detecting fraudulent activity. This article delves into the history, current trends, and future prospects of fraud detection in finance, with a focus on how high-interest rates play a critical role in shaping fraud prevention mechanisms.
1. Introduction to Fraud in Finance
Fraud in finance refers to any illegal act carried out with the intent of obtaining financial gain through deception. These activities include embezzlement, misrepresentation, identity theft, money laundering, and more. The financial sector, being one of the most critical pillars of the economy, has always been a target for fraudsters.
With advances in technology, fraud has become more sophisticated. Traditional methods of detection are no longer sufficient to tackle the more complex fraud schemes that modern financial institutions face. As a result, the evolution of fraud detection techniques is necessary to ensure the stability of financial systems.
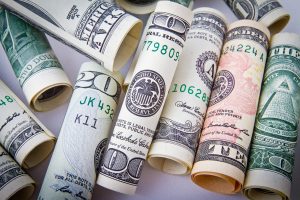
2. Historical Context of Fraud Detection
In the past, fraud detection primarily relied on manual processes. Bank tellers, auditors, and financial controllers were the first line of defense. The approach was reactive, with institutions only detecting fraud after it had occurred. While this method was somewhat effective for simpler fraud schemes, it proved inadequate as financial operations expanded globally.
Manual processes were not only time-consuming but also prone to human error. As a result, fraudsters found new ways to exploit weaknesses in the financial system. The increased use of credit and debit cards in the late 20th century saw a spike in identity theft and credit card fraud, pushing financial institutions to seek more advanced detection systems.
3. Technological Advancements in Fraud Detection
The rise of technology, particularly the development of computers and data analytics, revolutionized the field of fraud detection. In the 1990s and 2000s, fraud detection systems began to incorporate automation, which allowed for faster and more accurate detection. The following are some key technological advancements that have significantly impacted fraud detection in finance:
a. Data Analytics
With the growing availability of data, financial institutions started using data analytics to identify fraud patterns. By analyzing large datasets, banks and other financial entities could detect irregularities that might indicate fraud. Data analytics tools could flag suspicious transactions based on historical data, helping institutions take preventive measures before the fraud occurred.
b. Artificial Intelligence (AI) and Machine Learning (ML)
AI and ML have further transformed fraud detection methods. These technologies use algorithms to learn from historical data and predict future fraud patterns. With each iteration, these systems become more effective in identifying fraudulent activity, even as fraudsters develop new tactics.
AI and ML are especially useful in detecting anomalies in transaction data. For instance, if a customer who regularly makes small purchases suddenly withdraws a large sum of money, an AI-powered system can flag the transaction as potentially fraudulent.
c. Blockchain Technology
Blockchain technology offers another layer of security in fraud detection. By creating an immutable ledger of transactions, blockchain reduces the likelihood of tampering. While still in its early stages of adoption, blockchain is already showing promise in enhancing fraud prevention mechanisms, particularly in areas like identity verification and cross-border payments.
4. Impact of High-Interest Rates on Fraud Detection
High-interest rates can have a profound effect on the behavior of financial markets and participants. When interest rates rise, borrowing becomes more expensive, and this can lead to an increase in fraud attempts, particularly in sectors like lending, credit, and mortgages.
a. Increased Risk of Loan Fraud
With high-interest rates, individuals and businesses may face financial strain, prompting some to engage in fraudulent activities to secure loans. For example, applicants may provide false financial statements to qualify for loans they cannot afford, which is a common form of loan fraud. Financial institutions must remain vigilant during periods of high-interest rates, using enhanced fraud detection techniques to scrutinize loan applications more carefully.
b. Investment Fraud
High-interest rates also shift the focus of investors, who might seek higher returns in riskier assets. Fraudsters can exploit this by promoting fraudulent investment schemes, particularly those offering returns that seem to outpace the rising interest rates. Institutions need to implement sophisticated fraud detection tools to identify and block such schemes.
c. Heightened Regulatory Scrutiny
High-interest rate environments typically lead to increased regulatory scrutiny, as governments and financial watchdogs aim to prevent market instability. This has driven the evolution of fraud detection systems that are better equipped to ensure compliance with regulatory requirements, while simultaneously detecting any fraudulent activity.
5. Current Trends in Fraud Detection
The present landscape of fraud detection in finance reflects a convergence of multiple technologies and approaches. Some key trends include:
a. Biometric Verification
To combat fraud, financial institutions are increasingly using biometric verification, such as fingerprint and facial recognition. This adds a layer of security that makes it harder for fraudsters to use stolen identities to commit fraud.
b. Behavioral Analytics
Behavioral analytics involves monitoring how users interact with financial systems. For example, an individual’s typical pattern of online banking activity might be analyzed, and any deviation from this behavior could trigger an alert. This helps detect fraud in real time, even before any financial loss occurs.
c. Collaboration Between Institutions
Financial institutions are now working more closely with each other and with government agencies to combat fraud. Information sharing about fraud attempts and successful fraud schemes helps to develop better fraud detection methods across the industry. This collaborative effort is essential in tackling more organized forms of financial crime, such as money laundering.
6. Future of Fraud Detection
The future of fraud detection in finance is likely to be dominated by AI, machine learning, and blockchain technologies. As these systems become more sophisticated, financial institutions will be able to detect and prevent fraud in real-time, reducing the likelihood of large-scale financial losses.
a. Real-Time Fraud Detection
In the future, financial institutions will increasingly rely on real-time fraud detection systems that monitor transactions as they happen. With AI and ML continuing to evolve, these systems will become more adept at identifying even the most subtle forms of fraud.
b. Enhanced Regulatory Compliance
As regulations become stricter, particularly during periods of high-interest rates, financial institutions will need to ensure their fraud detection systems are compliant with evolving legal requirements. This will likely lead to the development of more comprehensive fraud detection solutions that incorporate regulatory checks as part of the fraud detection process.
7. Analysis Table: Key Factors in Fraud Detection Evolution
Factor | Historical Context | Modern Developments | Future Prospects |
---|---|---|---|
Technology | Manual detection systems | AI, ML, Data Analytics | Real-time detection with advanced AI |
Data Availability | Limited data, mostly manual records | Big data analytics | AI-powered predictive analytics |
Fraud Detection Techniques | Reactive fraud detection | Proactive fraud prevention systems | Self-learning fraud detection mechanisms |
Collaboration | Minimal information sharing | Industry-wide collaborations | Global data sharing for fraud prevention |
Interest Rates Impact | Mild influence during low-interest rates | High-interest rates increase fraud risks | Interest rate-sensitive fraud detection tools |
8. Comparative Table: Traditional vs. Modern Fraud Detection
Aspect | Traditional Fraud Detection | Modern Fraud Detection |
---|---|---|
Method | Manual audit and verification | Automated, AI and ML-driven systems |
Speed | Reactive, slow detection | Proactive, real-time detection |
Accuracy | Prone to human error | High accuracy with AI |
Scalability | Limited to small-scale operations | Highly scalable across global operations |
Adaptability | Slow to adapt to new fraud schemes | Quick adaptation using machine learning |
9. Conclusion
The evolution of fraud detection in finance reflects the changing dynamics of the financial world. As fraudsters become more sophisticated, financial institutions must continue to invest in advanced detection technologies to stay ahead. High-interest rates, in particular, bring added challenges, making it critical for institutions to enhance their fraud detection strategies. With the integration of AI, machine learning, and blockchain, the future of fraud detection holds the promise of real-time, efficient, and highly accurate systems capable of safeguarding the financial system.